Using model simulations to support monitoring - Methods&Techniques
Contents
Introduction
Numerical models offer information about marine circulation, the atmospheric state (radiation, temperature and wind, for instance) and other sea parameters like wave action, even for places and variables for which measurements do not exist. Such information is very useful for supporting monitoring activities. Benefits of combining observations and simulations of the physical environment arise in two respects. Firstly, high resolution model simulations allow for the interpretation of continuously observed data in a more regional context. Secondly, long term model simulations of the physical environment are needed for a proper interpretation of long term observations in the presence of natural variability. We will give examples for these two types of application.
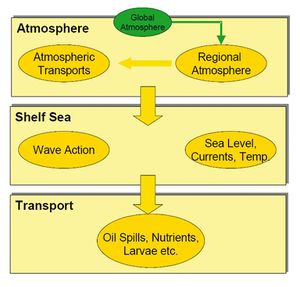
Data from coastDat
For the applications presented below we used data from coastDat that originated from the EU funded project HIPOCAS (Hindcast of Dynamic Processes of the Ocean and the Coastal Areas). The data consistently combine atmospheric simulations with simulations of currents, sea levels and waves. Fig. 1 outlines how these data are related to each other. Current and sea level fields were produced by running the finite element model TELEMAC (Hervouet and van Haren, 1996[1]). For a more detailed discussion of the model setup the reader is referred to Plüß (2004[2]). In the applications summarised below the data from coastDat were employed in different ways. The first approach is to aggregate detailed information on the North Sea flow regime in terms of characteristic flow patterns. The second is to link a tool for Lagrangian transport simulations to the fields from coastDat.
Flow patterns in the German Bight
To be in line with the concept of mass balance, we chose water flow (product of velocity and total water depth) as the relevant physical variable to be related to biological observations. Fourier analysis was applied to remove all variations in the model data that are related to astronomically prescribed frequencies with time scales up to one year. The filtered residual data contain neither tidal nor long term seasonal cycles. Hourly fields of residual water flow were then subjected to Empirical Orthogonal Function (EOF) analysis (von Storch and Zwiers, 1999[3]), a standard statistical technique also known as Principal Component Analysis (PCA). Two leading spatial patterns of water transport variability in the inner German Bight were identified (Fig. 2). They explain 70 % and 17 %, respectively, of total variance. Changing amplitudes of these patterns are represented by the time series of principal components (PCs, Fig. 3).
Lagrangian trajectories (PELETS-2d)
PELETS-2d (Programme for the Evaluation of Lagrangian Ensemble Transport Simulations) is a toolbox for Lagrangian drift modelling based on fields from coastDat. Particle trajectories calculated from the marine circulation fields may or may not take into account wind drift as an additional forcing factor. The latter is needed when dealing with oil slicks or drifting material, for instance. PELETS-2d has been designed in a way that it can easily be linked to the outputs of different hydrodynamic models, including models that are based on irregular grids. The important feature of PELETS-2d is that it allows for an efficient production of ensemble simulations. A toolbox for the evaluation of such simulations is provided. Source and target regions may be defined in order to balance transport rates and travel times, for instance, between different subregions of an area of interest. Results of ensemble simulations may be studied in terms of composites for various original or derived variables. Patterns of variations can easily be analysed by the application of EOF analysis.
References
- ↑ Hervouet, J. M. & van Haren, L. (1996). TELEMAC2D Version 3.0 Principle Note. Rapport EDF HE-4394052B, Electricité de France. Chatou Cedex: Département Laboratoire National d’Hydraulique.
- ↑ Plüß, A. (2004). Das Nordseemodell der BAW zur Simulation der Tide in der Deutschen Bucht. Die Küste 67, 83-127.
- ↑ von Storch, H. & Zwiers, F. W. (1999). Statistical Analysis in Climate Research. Cambridge: Cambridge University Press.
Please note that others may also have edited the contents of this article.
|
Please note that others may also have edited the contents of this article.
|
Please note that others may also have edited the contents of this article.
|